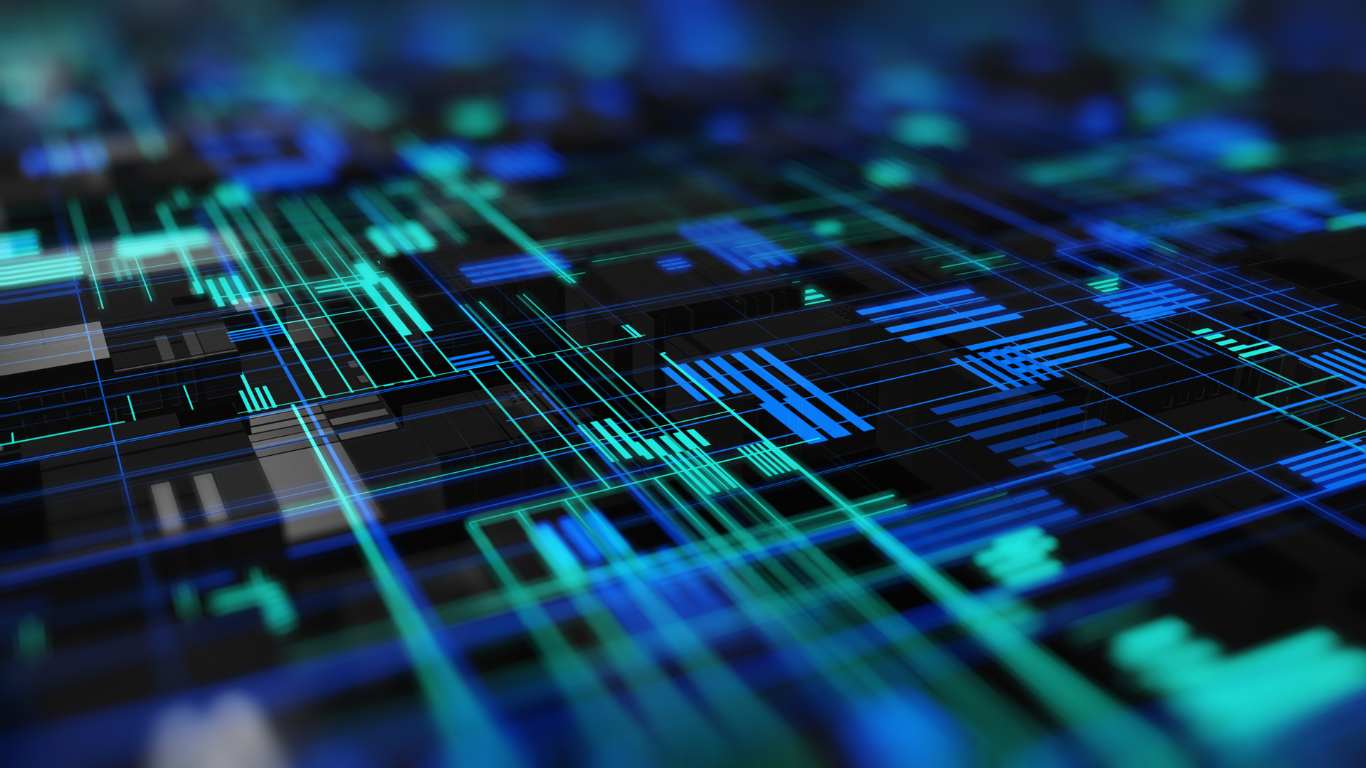

Read Time:
10
Minutes
Innovation @ Ntegra
March 6, 2025
Enhancing AI with Precision: The Evolution and Impact of Retrieval-Augmented Generation
Large Language Models (LLM) have transformed Generative AI in recent years. We met OpenAI in May 2022, as part of Ntegra's Silicon Valley Tech Summit (SVTS), 6 months before ChatGPT was unveiled to the public and LLMs became a common productivity tool for both enterprise and individual users alike.
The broad benefit of LLMs is that they are particularly good at generating meaningful strings of text having been trained on masses of publicly available data. However, these are general models that perform well on ordinary content generation tasks. General models have limited or no access to specific “in-house” data as part of their training for example, they do not have access to your SharePoint repositories or document stores. In addition, LLMs may struggle to handle rapidly changing or dynamic information, as they are trained on static datasets (Ribas, M. 2024).
Tailored for particular industries or even specific business use cases, customised large language models undergo training on a dataset specifically relevant to a distinct usage. Consequently, these customised LLMs can produce content that more accurately reflects the requirements (and information) of the business.
Whilst some industry specific LLMs are available (e.g. BloombergGPT, or Med-PaLM), most organisations cannot create entirely custom LLM since the computational resources to create them are prohibitively expensive. Constructing custom LLMs demands substantial time and expertise, involving intensive activities like data collection, annotation, model refinement and validation. In addition, engaging with domain specialists and machine learning professionals may escalate development costs.
Functionally, there has been a clear desire to combine the availability (and cost-effectiveness) of a general LLM with the improved accuracy, relevance and characteristics of a custom LLM.
This is where Retrieval-Augmented Generation (RAG) is becoming a favoured technique to provide improved relevance and accuracy. The RAG approach has developed from a research paper in 2020, led by Facebook researcher Patrick Lewis, aimed at exploring “a general-purpose fine-tuning recipe” (Piktus, A., et al. 2021).
Traditionally, retrieval models excel at recalling relevant information from large data stores, such as databases, documents or web sources, based on user queries or input. However, these retrieved snippets often lack coherence and context when directly presented to users. On the other hand, generation models, such as LLMs, possess the ability to generate human-like text but may lack access to real-world knowledge or context. RAG combines the strengths of both retrieval and generation models, enhancing how AI systems understand, process and generate human-like responses across various applications.
Therefore, at its core, RAG integrates the proficiency of retrieval models in accessing vast repositories of knowledge with the creative capacity of generation models to produce responses that are not only fluent but also deeply informed and contextually relevant.
Put simply, RAG boosts the LLM performance, by ensuring it references a trusted knowledge base outside of its training data sources before generating a response.
HOW DOES RAG WORK?
The original 2020 paper describes “a general-purpose fine-tuning recipe for retrieval-augmented generation (RAG) — models which combine pre-trained parametric and non-parametric memory for language generation”.
Parametric memory allows AI models to remember and access relevant information across different tasks or contexts by encoding it into a set of learnable parameters. These parameters capture the essential features or representations of the input data, allowing the model to generalise and make informed decisions based on past experiences. Parametric memory is developed from the underlying training process.
Non-parametric memory uses explicit data structures or mechanisms to store and retrieve information. External memory refers to dedicated storage units or data structures that are separate from the model's parameters. Examples include traditional data structures like arrays, lists or hash tables, as well as more advanced memory architectures like memory networks or pulling from APIs.
These performance improvements are achieved through a two-stage process. First, the retrieval component selects relevant information from a knowledge source and then the generation component synthesises this information into a coherent response tailored to the user's query or context.

THE POPULARITY OF RAG
The popularity of RAG can be attributed to its versatility and effectiveness across a wide range of AI applications. One of the primary areas where RAG has gained prominence is in Natural Language Processing (NLP), particularly in conversational agents, chatbots and virtual assistants. These AI-driven systems rely on understanding user queries and providing relevant responses in natural language. By leveraging RAG techniques, chatbots can retrieve information from diverse sources such as knowledge bases, FAQs or the internet and generate responses that are not only accurate but also contextually appropriate, enhancing user satisfaction and engagement.
Furthermore, RAG has proven to be invaluable in knowledge-intensive domains such as healthcare, finance and legal services. AI systems augmented with RAG capabilities can analyse large volumes of textual data, retrieve relevant information from medical records, financial reports or legal documents and generate insights or recommendations that aid decision-making processes. For instance, in healthcare, RAG systems can assist doctors in diagnosing illnesses, suggesting treatment options or providing patient education materials based on the latest medical research and guidelines.
WHAT TECHNOLOGY TRENDS DOES RAG DRIVE?
Like all AI approaches, RAG relies on various foundational components and strategies including robust algorithms, quality data management for effective retrieval, efficient computational resources and skilled human oversight. The outputs of such systems need to be managed and assured such that they can be trusted to assist complex business decision making.
As such, we see trends in the continued development of enterprise and developer strategies aimed at optimising different component parts of RAG and other AI approaches. This appears to be a significant trend at the moment.
UNDERPINNING DATA MANAGEMENT
Unstructured specialises in converting natural language data from its raw form into a format ready for machine learning applications. They provide open-source libraries and APIs to create custom pre-processing pipelines for tasks such as labelling, training and deploying machine learning models.
Select Star is a fully automated data discovery platform that helps everyone find, understand and use company data. You can understand column and table-level relationships in seconds with data lineage, catalogue and automated documentation.
IMPROVED QUERY AND RETRIEVAL TOOLS
SunDeck is the creator of an SQL orchestration platform that sits between end-users (ETL, Analysts, Data Science tooling) and underlying SQL-based engines such as databases and distributed computing systems. The platform will enable functionalities such as improved cost management for SQL queries as a wedge to the market but will roll out additional functionalities and apps such as monitoring & responding, intelligent routing, privacy protection and query results caching over time.
ENTERPRISE ASSURANCE AND SECURITY OF AI
Securiti offers a centralised platform that enables the safe use of data and GenAI. They focus on mitigating complex security, privacy and compliance risks.
Vijil is building the trusted layer for Generative AI across cloud, on-prem and edge systems. Vijil believes there is a need for an ‘independent’ peer-reviewed trust score and methodology that is independent of the model creator.
Fiddler's mission is to allow businesses of all sizes to unlock the AI black box and deliver transparent AI experiences to end-users. They enable businesses to build, deploy and maintain trustworthy AI solutions. Fiddler's next-generation ML Model Performance Management platform empowers data science and AI/ML teams to validate, monitor, explain and analyse their AI solutions.
MANAGEMENT LAYER FOR AI
Martian, an AI research specialist team, is on a mission to build advanced AI tooling by understanding intelligence algorithms. They are inspired by "The Martians," a group of Hungarian-American scientists and aim to crack open AI's "black box" to harness modern super intelligence.
NEW FOUNDATIONAL APPROACHES AND RAG 2.0
While some start-ups aim to improve existing AI methods and techniques, some companies continue to conduct deep research and seek to develop new foundational models.
Contextual AI is building the next generation of Large Language Models, geared specifically for enterprise use cases. Contextual AI co-founder and CEO Douwe Kiela was part of the team that wrote the original RAG paper in 2020. Now he and his Contextual AI team are looking to enhance the original RAG approach, to create what they call Contextual Language Models (CLM).
Whilst existing RAG approaches augment existing language models, Contextual say these “Frankenstein’s monsters” have individual components that work but are not optimised as a whole.
A recent blog post describes how the Contextual AI RAG 2.0 approach pre-trains, fine-tunes and aligns all components as a single integrated system, back propagating through both the language model and the retriever to maximise performance (www.contextual.ai/introducing-rag2).
“CLMs outperform strong RAG baselines based on GPT-4 and the best open-source models by a large margin, according to our research and our customers.”
CONCLUSION
In conclusion, retrieval-augmented generation (and recent proprietary derivatives) represents a significant advancement in the field of AI, transforming how AI tools understand, process and generate accurate responses across various applications.
By combining the strengths of retrieval and generation mechanisms, RAG systems enable AI to produce contextually relevant and informative responses, enhancing user experience and engagement in conversational agents, content creation, knowledge-intensive tasks and beyond.
As RAG continues to evolve, we also see developments in enterprise and developer tools aimed at continuing to optimise competing AI approaches and underpinning technologies.
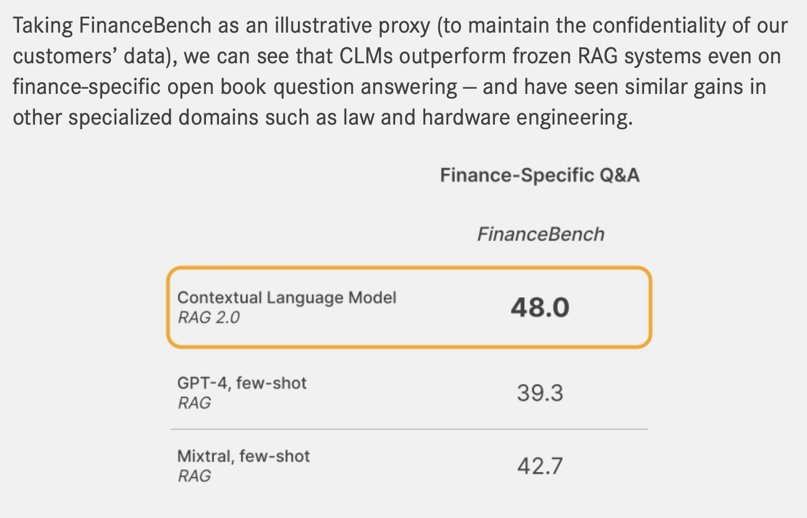
Entirely custom LLMs continue to be out of reach for many enterprises until more efficient training methods, model architectures and computational resources bring down costs and the complexity of building LLMs from the ground up.
In the meantime, it is clear that solutions that combine the speed and efficiency of general purpose LLMs with the ability to increase relevance for specific use cases and business needs will continue to prove valuable.
Authored by Ben Parish, Head of Innovation